Artificial intelligence to be used for the detection of common eye disease
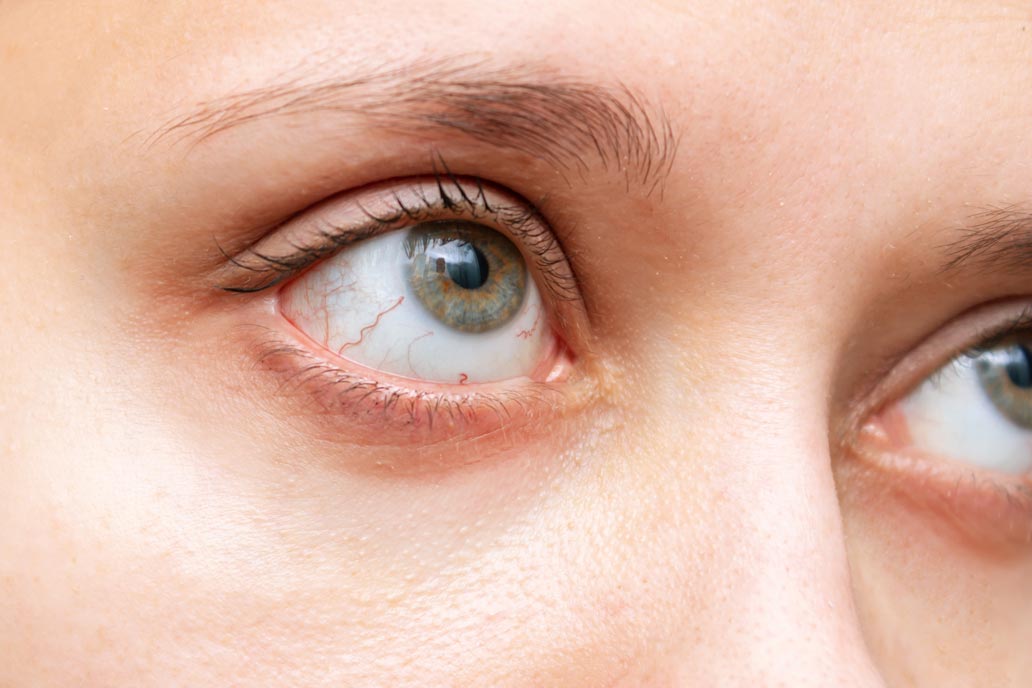
Dry Eye Disease (DED) is one of the more common eye diseases, affecting up to 30% of the world’s population. This disease can affect many different types of people and can wind up being a great hindrance to their overall quality of life. Early screening and prognosis is vital to the patient’s progression with the disease. However, this can be difficult. In this study, researchers aim to use artificial intelligence (AI) to aid in early screening and prognosis of DED. Not only can the use of AI make screening more accessible for individuals, but it can also aid patients in personalized therapeutic intervention.
Researchers published their results in Big Data Mining and Analytics on April 22.
DED can affect a wide array of people, including those who wear contact lenses, makeup, stay up late, look at screens for a long time and are over 30 years old. Symptoms of this disease are dry eyes, irritation and burning, tears, eye fatigue and pain. One can easily see how this disease has the potential to drastically impact a large portion of the modern world’s population. Here is where the combined efforts of ophthalmic disease detection and the world of computer scientists and engineers can help.
“By addressing challenges, imparting insights, and delineating future research pathways, it contributes substantially to the advancement of ophthalmic disease detection through sophisticated technological modalities,” said Mini Han Wang, author and researcher.
There are seven facets to this AI-based disease detection. Timely intervention via the AI screening process and correct prognosis is the first part. The use of exhaustive surveys for DED through AI is another, and this is a supporting principle to ensure a level of thoroughness and trustworthiness throughout the process. A systematic approach follows, as well as the marriage of computer science and engineering with ophthalmology. Then, the standards for DED detection must be devised and upheld for future researchers and practitioners, which will naturally lead to the advancement of the field. Finally, all the research, methodologies and tools must be compiled so researchers, scholars and practitioners can have all of the information currently out there available to them.
While the ophthalmologists set the guidelines regarding the framework of the disease and flags for diagnosis, the AI does a lot of the heavy lifting. Ideally, this AI would use images and videos taken from a user’s cell phone to help reach users across the world. The AI can then utilize these images, as well as risk factors in the patient’s life, to make a smart and well-informed prognosis. Further, AI continuously learns and can help propel research forward by contributing to predictive models for DED.
The use of AI detection for DED holds a lot of promise, especially considering the risk factors are often normal activities in many people’s everyday lives. To make the detection methods accessible enough and accurate enough, further research needs to be done.
“However, there are still challenges for engineers to select the diagnostic standards and combinations of different types of datasets. By using trustworthy algorithms, images and videos captured from phones for accessibility purposes, a holistic approach to healthcare for early screening is possible,” said Wang.
With continued testing and collaboration between engineers and ophthalmologists, there is great potential for this method of testing to be useful in contributing to early screening of DED and subsequent therapeutic actions taken for the patient to reduce a worsening condition or to recover some quality of life.
Mini Han Wang and Xiangrong Yu of the Zhuhai People’s Hospital with Mini Han Wang also of the Department of Ophthalmology and Visual Sciences at the Chinese University of Hong Kong, The Faculty of Data Sciences at City University of Macau and the Department of big data at the Zhuhai Institute of Advanced Technology at the Chinese Academy of Sciences, Lumin Xing of the First Affiliated Hospital of Shandong First Medical University, Yi Pan of the Shenzhen Institute of Advanced Technology Chinese Academy of Sciences, Feng Gu of the College of Staten Island at the City University of New York, Junbin Fang at the Department of Optoelectronic Engineering at Jinan University, Chi Pui Pang, Kelvin KL Chong, Carol Yim-Lui Cheung and Xulin Liao of the Department of Ophthalmology and Visual Sciences at The Chinese University of Hong Kong, Xiaoxiao Fang with the Zhuhai Aier Eye Hospital, Jie Yang of the College of Artificial Intelligence at Chongqing Industry and Trade Polytechnic, Ruoyu Zhou and Wenjian Liu with the Faculty of Data Science at City University of Macao, Xiaoshu Zhou with the Centre for Science and Technology Exchange and Cooperation between China and Portuguese-Speaking Countries, and Fengling Wang with the School of Artificial Intelligence at Hezhou Univeristy contributed to this research.
The National Natural Science Foundation of China Natural, the Shenzhen Key Laboratory of Intelligent Bioinformatics, the Shenzhen Science and Technology Program, the Guangdong Basic and Applied Basic Research Foundation, the Zhuhai Technology and Research Foundation, the Project of Humanities and Social Science of MOE, the Science and Technology Research Program of Chongqing Municipal Education Commission and the Natural Science Foundation of Chongqing China made this research possible.